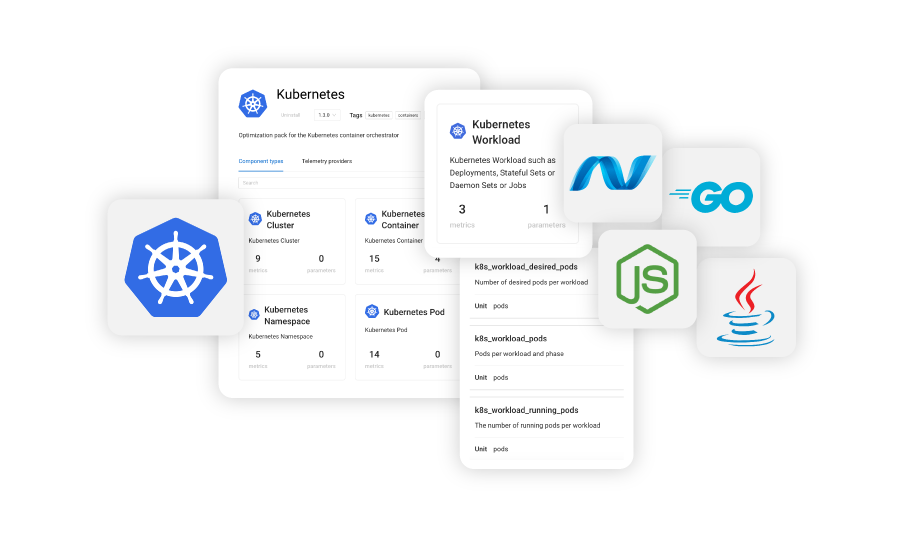
Full Stack
Optimize pod resources and applications, together
Akamas supports the range of technologies across enterprise cloud-native stacks, from Kubernetes to runtimes like JVM, Node.js, .NET and Golang settings, and takes into account their interdependencies.
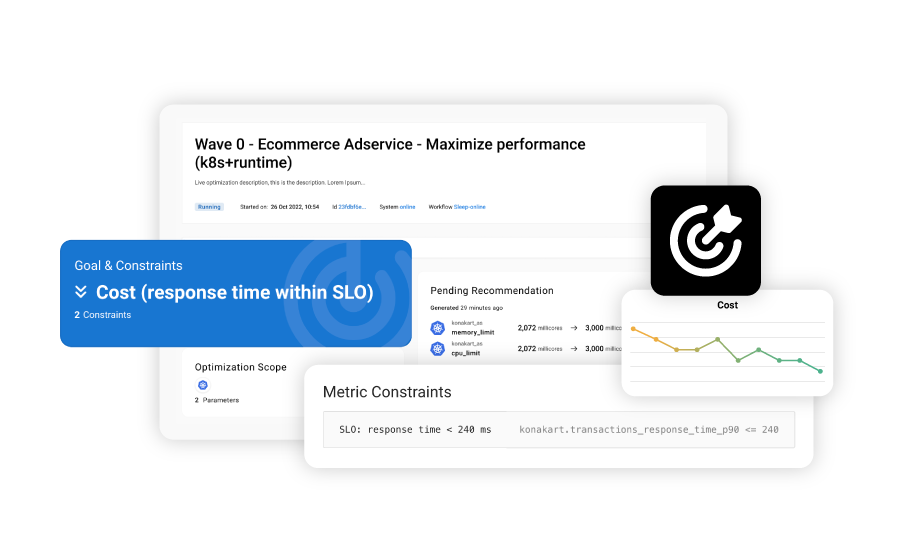
GOAL-Oriented
Balance cost targets and performance optimization goals
Akamas is designed to achieve complex user-defined goals. Users can set resource usage, application performance, and response time goals, while respecting latency, error rate and SLO constraints.
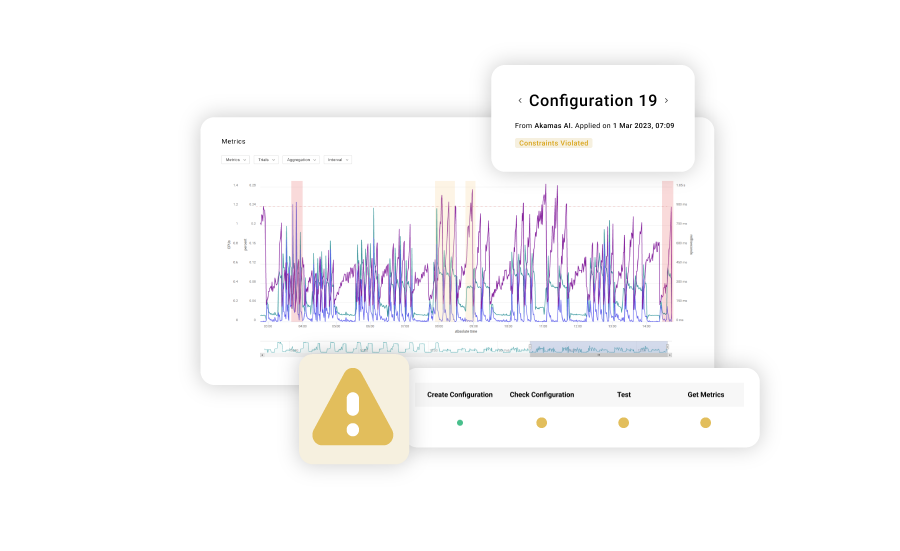
Safe
Prevent dangerous application configurations
Akamas AI learns continuously from system and application signals, such as response time and error rates, finding stack configuration sets that prevent downstream issues such as out-of-memory errors.